PROJECTS
PhD Thesis
​
​
My PhD was focused on how brains learn and recalls procedural memories or motor skills.
​
These are a specialised kind of animal behaviour, based on movement patterns, learned for a particular environmental context and performed with exceptional spatial and temporal precision.
​
These behaviours are ubiquitous and range from tying a shoelace or typing a password up to cognitively complex behaviours such as producing speech.
​
My investigation considered two distinct but overlapping themes.
-
Firstly, I explored thalamic and striatal circuits which support skill learning and production online: during wakeful behaviour.
-
In the second part of my thesis, I examined the processes which support the function of these circuits offline: during rest or sleep.
-------------------------
​
​
​
​
​
​
​
​
​
​
​
​
​
​
​
​
​
​
​
​
​
During my PhD defence I gave an open talk explaining my work
​
-------------------------
​
​
​​Thesis: Subcortical circuits for procedural learning and memory
Symposia
2020 - Neural Interfaces for Neurobiological Insights​
The intersection between brain-machine interfaces, AI and our understanding of the brain.
Speakers:
Aron Batista (university of Pittsberg)
Amy Orsborn (University of Washington)
Jaun Gallego (Imperial College London)
Kelly Clancy (Deepmind)
Edward Chang (UCSF)
Tamar Makin (University College London)
Danielle Clode (University College London)
Industry talks from Icibici & BIOS
---------------------------------------------------------------------------------------
2019 - Generalisation and abstraction
Neural underpinnings of higher cognitive functions
Speakers:
Tim Behrens (Oxford/University college London)
Stefano Fusi (University of Columbia)
Irina Higgins (Deepmind)
Alla Karpova (hhmi Janelia)
EEG-splatting
An interdisciplinary project undertaken with technical artist Jenn Leung & software engineer Daniel Humphries that explores the intersection of neuroscience, machine learning, and digital arts. My role in this project was as a neural interface engineer.
This project aims to demystify AI in the context of brain-machine-interfaces using electroencephalogram (EEG) signals recorded live from participants to drive artistic visualisations. Filtered LFP signals are decoded and the resultant state dynamics are fed into the hidden layers of a 3D model (NeRF) and visualised in Unreal Engine.
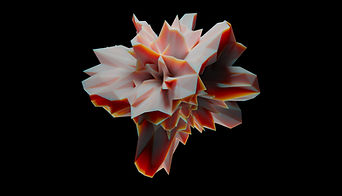